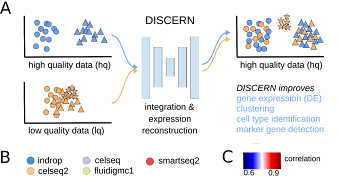
Single cell sequencing provides detailed insights into biological processes including cell differentiation and identity. While providing deep cell-specific information, the method suffers from technical constraints, most notably a limited number of expressed genes per cell, which leads to suboptimal clustering and cell type identification. We developed DISCERN, a novel deep generative neural network that reconstructs missing single cell gene expression using a reference dataset. DISCERN based expression inference results in greatly improved cell clustering, cell type and activity detection, and can lead insights into the cellular regulation of disease. For example, we used DISCERN to detect two cell types with a potential role in adverse COVID-19 outcome. DISCERN can be easily integrated into existing single cell sequencing workflows and readily adapted to enhance various other biomedical data types.
Stay In Touch